Low-Overhead and Fully Automated Statistical Debugging with Abstraction Refinement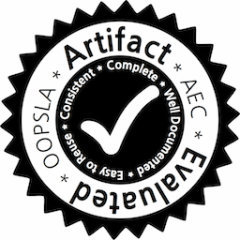
Cooperative statistical debugging is an effective approach for
diagnosing production-run failures. To quickly identify failure
predictors from the huge program predicate space, existing techniques
rely on random or heuristics-guided predicate sampling at the user
side. However, none of them can satisfy the requirements of low cost,
low diagnosis latency, and high diagnosis quality simultaneously,
which are all indispensable for statistical debugging to be practical.
This paper presents a new technique that tackles the above
challenges. We formulate the technique as an instance
of abstraction refinement, where
efficient abstract-level profiling is first applied to the
whole program and its execution brings information that can pinpoint
suspicious coarse-grained entities that need to be refined.
The refinement profiles a corresponding set of fine-grained entities,
and generates feedback that determines what to prune and what to
refine next. The process is fully automated, and more importantly,
guided by a mathematically rigorous analysis that guarantees that our
approach produces the same debugging results as an exhaustive
analysis in deterministic settings.
We have implemented this technique for both C and Java on both single
machine and distributed system. A thorough evaluation demonstrates
that our approach yields (1) an order of magnitude reduction
in the user-side runtime overhead even compared to a sampling-based
approach and (2) two orders of magnitude reduction in the size of
data transferred over the network, completely automatically without
sacrificing any debugging capability.
Fri 4 NovDisplayed time zone: Amsterdam, Berlin, Bern, Rome, Stockholm, Vienna change
13:30 - 15:10 | Bug Detection Analysis and Model CheckingOOPSLA at Matterhorn 1 Chair(s): Ben Livshits Microsoft Research | ||
13:30 25mTalk | Finding Compiler Bugs via Live Code Mutation OOPSLA Chengnian Sun University of California, Davis, Vu Le Microsoft, Zhendong Su University of California, Davis DOI Media Attached | ||
13:55 25mTalk | Finding Resume and Restart Errors in Android Applications OOPSLA Zhiyong Shan University of Central Missouri, USA, Tanzirul Azim University of California at Riverside, USA, Iulian Neamtiu New Jersey Institute of Technology, USA DOI Pre-print | ||
14:20 25mTalk | Low-Overhead and Fully Automated Statistical Debugging with Abstraction Refinement OOPSLA Zhiqiang Zuo University of California, Irvine, Lu Fang University of California, Irvine, Siau-Cheng Khoo , Harry Xu University of California, Irvine, Shan Lu University of Chicago DOI Media Attached | ||
14:45 25mTalk | To Be Precise: Regression Aware Debugging OOPSLA DOI |